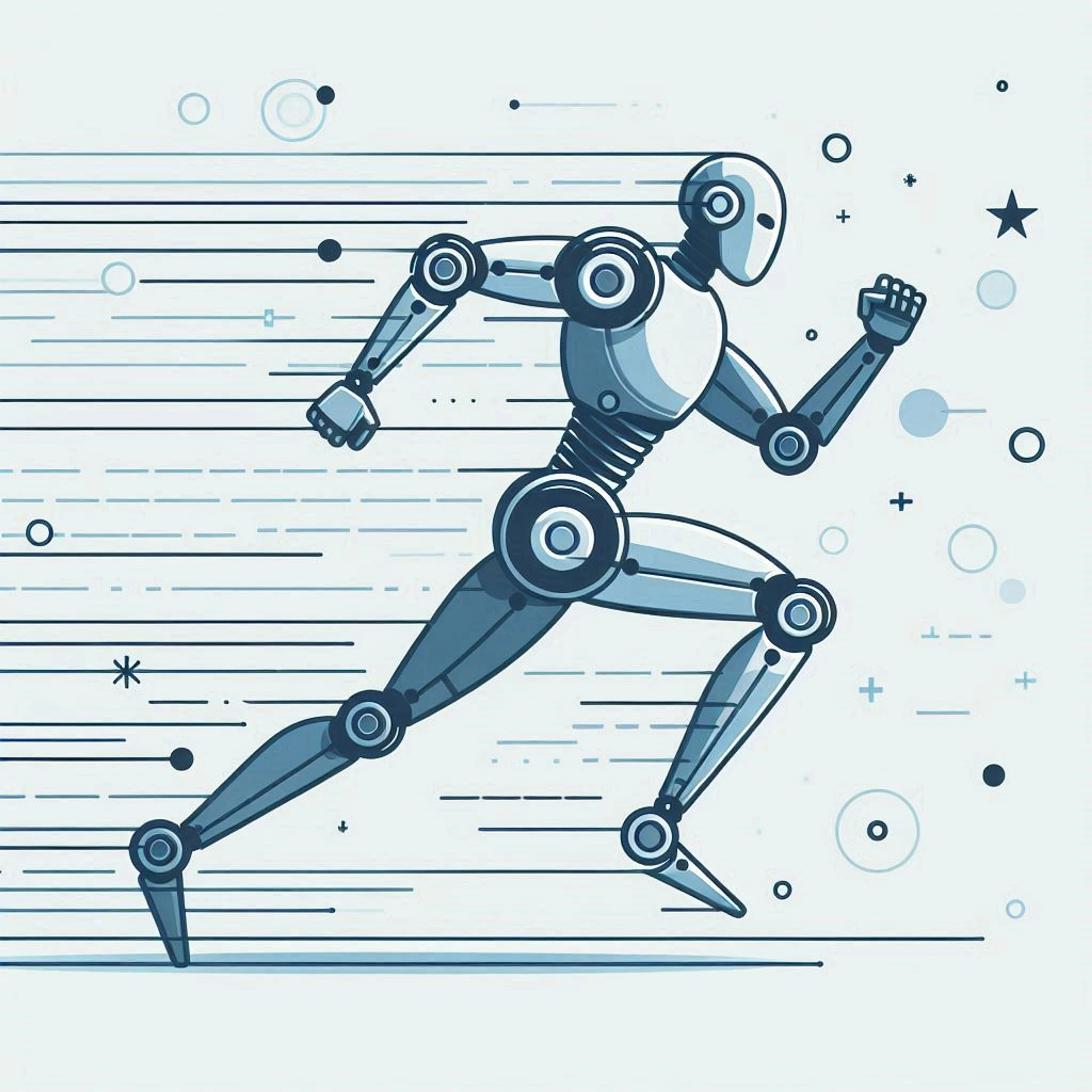
How to Host Your Own LLM with vLLM: The Cheapest, Fastest, and Easiest Way
Learn to host your own LLM with vLLM, deploying Llama 3 on any cloud quickly and cost-effectively. vLLM enables faster and more efficient inference, while Runhouse lets you deploy your code instantly to any cloud compute with no overhead.

Engineer @ 🏃♀️Runhouse🏠
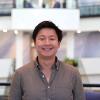
ML @ 🏃♀️Runhouse🏠
In late 2022, ChatGPT took the world by storm. At that point, only OpenAI’s hosted GPT-3 had the power to deliver quality answers. But by late 2023, there are now a variety of open-access models that match the performance of GPT-3. Of course, by most metrics, OpenAI’s GPT-4 is still the highest-quality model across almost every benchmark of readability, code generation, and reasoning. But not every task requires the best-of-the-best. Whether for cost control, data privacy, or customizability, there are many reasons why you might want to host your own large language model (LLM).
We will show you how to deploy the base Llama3 model that has been fine tuned for instruction following. Open source model sans fine tuning will not behave as we expect ChatGPT to, because they are naive to obeying a task like holding a chat conversation with a user. The Instruct fine-tuning was released by Facebook directly, but many models take a pretrained model and fine-tune even further for instruction following (obeying input requests), chat (question/answer), or writing code.
You can also fine tune yourself; we have a guide to fine-tuning using LoRA.
Why is this tutorial the cheapest, fastest, and easiest?
- Cheapest: There’s a lot of ways to measure cost, but in our opinion, the cheapest option is showing you how to write code that is portable and instantly deployable to any cloud compute without additional DevOps overhead. Runhouse enables you to directly send code to arbitrary compute, whether it’s Google Cloud as the example below or any other cloud provider.
- Fastest: We show you how to use vLLM, that produces outputs up to 24x faster than the standard Transformers library. vLLM has
Easiest: Here, we will show you how to load a model and serve it with normal Python, with <10 lines of code to serve in production infrastructure.
Code Example: Serve Llama3 with VLLM
You can find a full script available here: https://github.com/run-house/runhouse/blob/main/examples/llama3-vllm-gcp/llama3_vllm_gcp.py
Set up credentials and dependencies
For this exercise, you will need:
- A HuggingFace token to download the base Llama3 model. Make sure to accept terms and conditions on the Hugging Face model page so that you can access it.
- Access to compute - we will use an Google Cloud instance, but Runhouse can be used with any cloud compute you have access to, or even local compute if you have enough resources.
Run the following in Terminal or by command line to install Runhouse, and configure Google Cloud (GCP). You can change “gcp” to “aws” or to your cloud of choice.
$ pip install "runhouse[gcp]" asyncio $ gcloud init $ gcloud auth application-default login $ sky check $ export HF_TOKEN=<your huggingface token>
Define a Llama 3 model class
We import runhouse and asyncio because that's all that's needed to run the script locally. The actual vLLM imports are defined in the environment on the cluster in which the function itself is served.
Next, we define a class that will hold the model and allow us to send prompts to it. You'll notice this class inherits from rh.Module. This is a Runhouse class that allows you to run code in your class on a remote machine. Wrapping your code with this class is the only thing that is needed to make your Python ready to execute on remote compute and production ready.
Learn more in the Runhouse docs on functions and modules.
import asyncio import runhouse as rh class LlamaModel(rh.Module): def __init__(self, model_id="meta-llama/Meta-Llama-3-8B-Instruct", **model_kwargs): super().__init__() self.model_id, self.model_kwargs = model_id, model_kwargs self.engine = None def load_engine(self): from vllm.engine.arg_utils import AsyncEngineArgs from vllm.engine.async_llm_engine import AsyncLLMEngine args = AsyncEngineArgs( model=self.model_id, # Hugging Face Model ID tensor_parallel_size=1, # Increase if using additional GPUs trust_remote_code=True, # Trust remote code from Hugging Face enforce_eager=True, # Set to False for production use cases ) self.engine = AsyncLLMEngine.from_engine_args(args) async def generate(self, prompt: str, **sampling_params): from vllm.sampling_params import SamplingParams from vllm.utils import random_uuid if not self.engine: self.load_engine() sampling_params = SamplingParams(**sampling_params) request_id = random_uuid() results_generator = self.engine.generate(prompt, sampling_params, request_id) async for output in results_generator: final_output = output responses = [] for output in final_output.outputs: responses.append(output.text) return responses
Set up Runhouse primitives
The Python code we'll run is contained in an asynchronous function, main.
First, we create a cluster with the desired instance type and provider. Our instance_type here is defined as L4:1, which is the accelerator type and count that we need. We could alternatively specify a specific GCP instance type, such as g2-standard-8. Learn more in the Runhouse docs on clusters.
Next, we define the environment in `env` for our module. This includes the required dependencies that need to be installed on the remote machine, as well as any secrets that need to be synced up from local to remote. Passing huggingface to the secrets parameter will load the Hugging Face token we set up earlier. Learn more in the Runhouse docs on envs.
Finally, we define our module and run it on the remote cluster. We construct it normally and then call get_or_to to run it on the remote cluster. Using get_or_to allows us to load the exiting Module by the name llama3-8b-model if it was already put on the cluster. If we want to update the module each time we run this script, we can use to instead of get_or_to.
async def main(): gpu_cluster = rh.cluster( name="rh-l4x", instance_type="L4:1", memory="32+", provider="gcp", autostop_mins=30, # Number of minutes to keep the cluster up after inactivity ) env = rh.env( reqs=["vllm==0.2.7"], # >=0.3.0 causes Pydantic version error secrets=["huggingface"], # Needed to download Llama 3 from HuggingFace name="llama3inference", working_dir="./", ) remote_llama_model = LlamaModel().get_or_to( gpu_cluster, env=env, name="llama3-8b-model" )
Calling our remote function
We can call the generate method on the model class instance as if it were running locally. This will run the function on the remote cluster and return the response to our local machine automatically. Further calls will also run on the remote machine, and maintain state that was updated between calls, like self.engine.
prompt = "What are three great places to go on vacation?" ans = await remote_llama_model.generate( prompt=prompt, temperature=0.85, top_p=0.95, max_tokens=250 ) for text_output in ans: print(f"... Generated Text:\n{prompt}{text_output}\n")
Run the script
Finally, we'll run the script to deploy the model and run inference.
NOTE
Make sure that your code runs within a if __name__ == "__main__": block. Otherwise, the script code will run when Runhouse attempts to run code remotely.
if __name__ == "__main__": asyncio.run(main())
Your initial run of this script may take a few minutes to deploy an instance on GCP, set up the environment, and load the Llama 3 model. Subsequent runs will reuse the cluster and generally take seconds.
Advanced: Sharing and TLS endpoints
Runhouse makes it easy to share your module or create a public endpoint you can curl or use in your apps. Use the optional settings in your cluster definition above to expose an endpoint. You can additionally enable Runhouse Den auth to require an auth token and provide access to your teammates.
First, create or log in to your Runhouse account by running `runhouse login` from Terminal or command line.
Once you've logged in to an account, use the following lines to enable Den Auth on the cluster, save your resources to the Den UI, and grant access to your collaborators.
gpu_cluster.enable_den_auth() # Enable Den Auth gpu_cluster.save() remote_llama_model.save() # Save the module to Den for easy reloading remote_llama_model.share(users=["friend@yourcompany.com"], access_level="read")
OpenAI Compatible Server
By default, vLLM implements OpenAI's Completions and Chat API. This means that you can call your self-hosted Llama 3 model on GCP with OpenAI's Python library. Read more about this and implementing chat templates in vLLM's documentation.
Stay up to speed 🏃♀️📩
Subscribe to our newsletter to receive updates about upcoming Runhouse features and announcements.
Read More
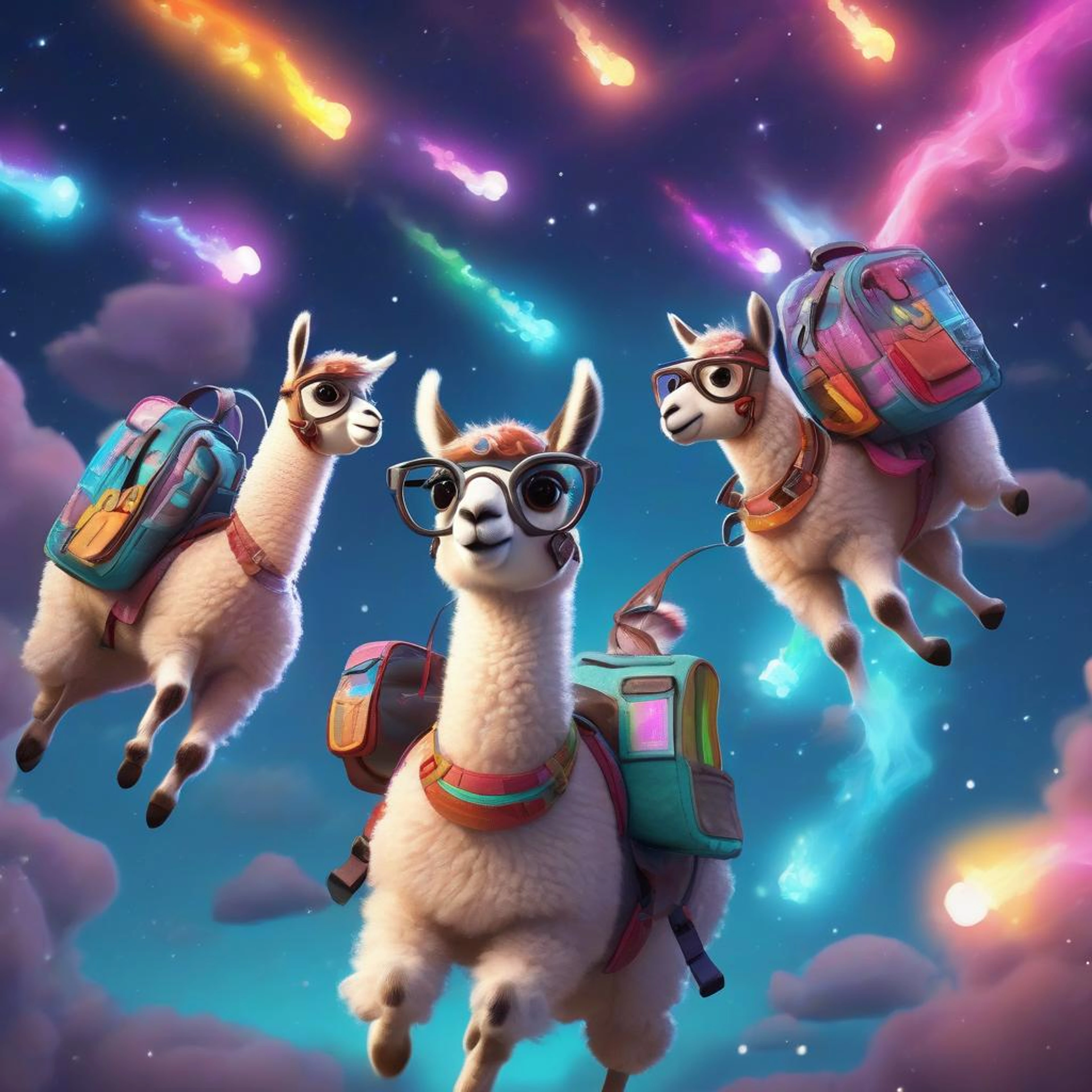
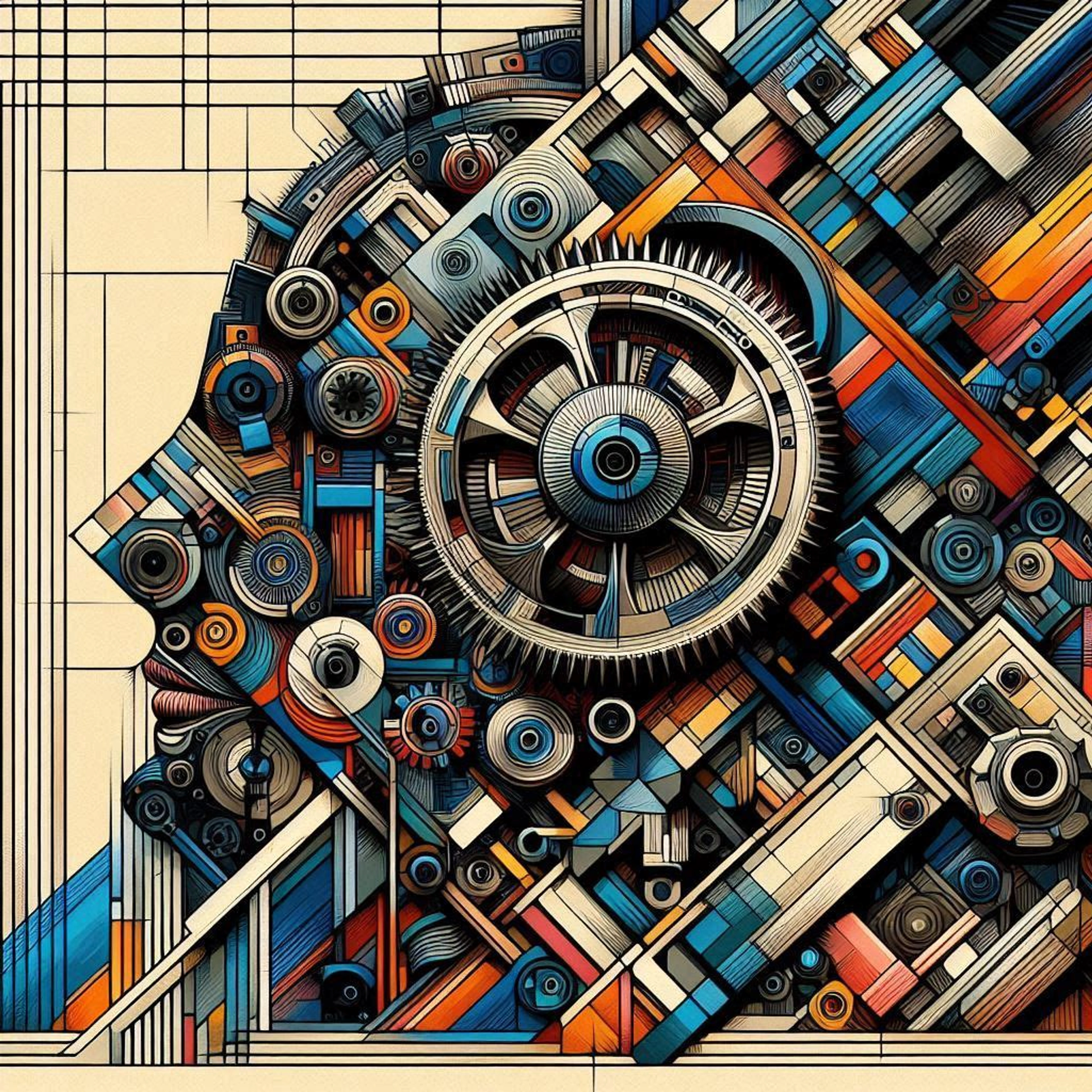